The value of data products sold externally will always be higher than for internal data products.
Last week, I wrote about what a data product is. I covered many types of product that could be both an internal or external data product. Companies use internal data products to optimise decision making by humans or through automation. External products are sold to customers of the company, and this is almost always a B2B context. It’s quite rare to sell data products to consumers, the exception in that post being something like Dark Sky.
Google’s Ads business generated $237Bn last year. This is about the size of the GDP of Qatar and is approximately 1% of US GDP. One business line of one company… You might say that this business is not a data product, but a marketing one - but I would disagree. Google collects huge quantities of data - more than any other company. They use this data to create a market where you can bid on terms or space to show your product or services.
The act of generating, maintaining and presenting what people can bid into is a data product in itself. It’s also possible to measure performance and choose to allow Google to spend your ad budget for you, where their algorithms will maximise value. If you are still in doubt that this is a data product, imagine if, instead of smart bidding, they made a new product which was a grid of graphs. The grid of graphs comes with recommendations to bid in a certain way. You could then take this information and use it to control ad spend. If you don't question this as a data product, then smart bidding is a data product too. It's a superior data product to the human-in-the-loop one.
Google's Ad business is a collection of data products - the data products of all data products. Even though it is under threat, it underpins the idea that “data is the new oil”. Investors looked at Google and wondered who else could make a huge business based on data. Facebook was the next company to do this. Amazon generate a huge data product to serve their own needs, a deep and broad knowledge of consumer preference.
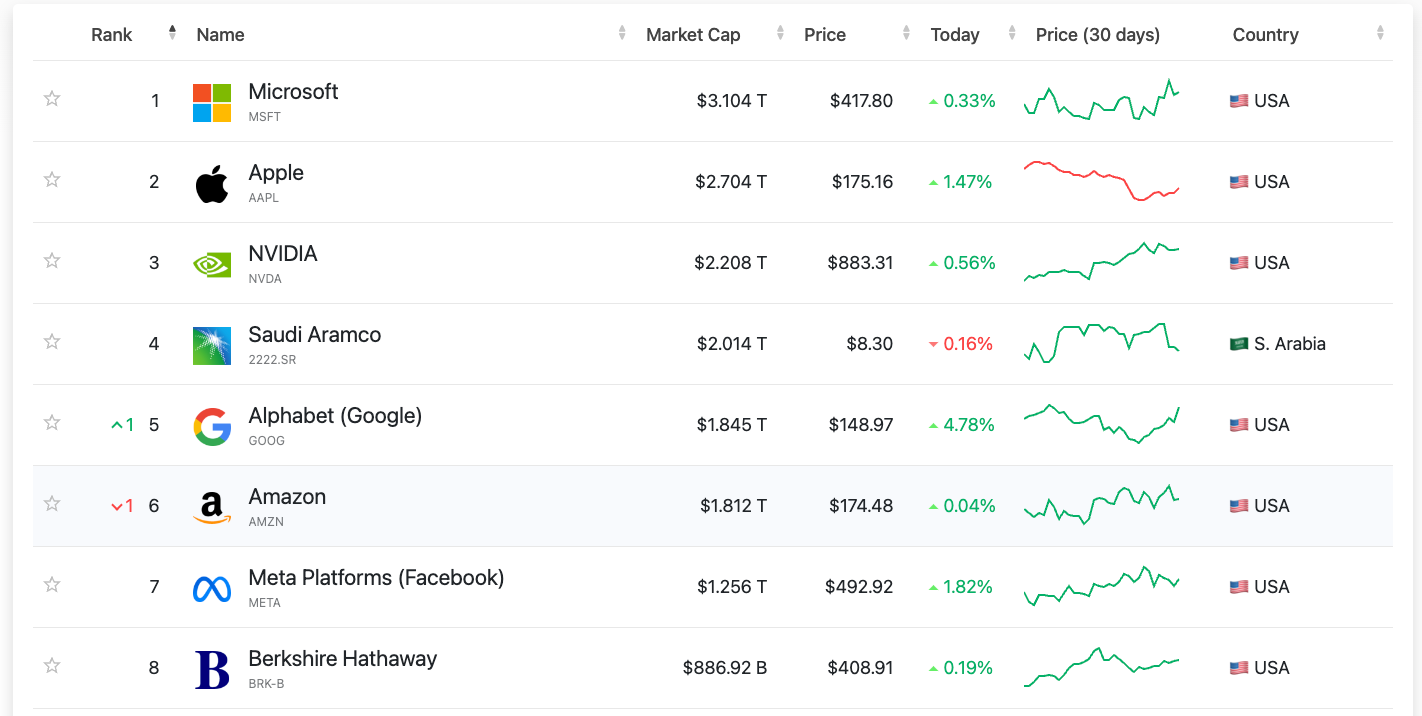
Google and Facebook sell a data product to other companies. Their moats are the intractable ways consumers use their services and provide them with the data that they use to make their data products. Where selling data products are a company’s business, you don’t hear them bemoaning data quality. You don’t hear them talking about data contracts, data mesh, data fabric or another supposed silver buzzword to their data problems. Their data problems have been solved, they have engineered it so. If they weren’t solved, they would get in the way of doing business.
Even on a smaller scale, we see companies whose business it is to sell a data product do a much better job of executing with data than others. A few weeks ago, I wrote about a presentation by someone at SpotOn, about their data engineering architecture. It wasn’t bemoaning data quality, it wasn’t crying out for data contracts, buzzwords like data mesh and fabric weren’t relevant at all. They already had a production system that worked, and they talked about how they made it even better, to deliver their data product even faster.
What a surprise, businesses who need to sell data products succeed with data. That doesn’t mean their internal BI data is perfect. It’s probably as good as anywhere else, unless it benefits from shared infrastructure with their data products. Where data is in the critical path of making money, success is prioritised, funded and engineered.
The flipside to this argument is that where data isn’t in the critical path of making money, it’s OK for it to be neglected… it’s not that important. Who cares if someone’s Tableau dashboard is wrong, if the consequence doesn’t affect the bottom line? They should never have had the dashboard in the first place.
Where data is not for sale in a data product, it is usually of lower quality because ensuring it is of high quality is not the top priority. The use of data might help optimise the business (but also might not).
Data’s Hierarchy of Value:
Build a data product for sale
Help your business grow
Help your business make and offer the right product
Accurately measure business performance
Underpinning all these activities is a data platform. As you can imagine, companies engaging in 1 will be more likely to invest more in data platform - it’s that much closer to the critical path of making money.
We know that Marketing teams have long had big budgets to invest in data - it’s somewhere data can easily pay its way and have a good ROI. 2 and 3 are very close and, depending on the organisation, may swap places. I’ve worked for a company where a similar amount was spent on Amplitude as my whole data stack and team.
Even though 4 is the lowest value data activity, it is the one that is most consistently present. It is the one that most people think of when they think of data work. The only part of a company that has budget for this kind of work, other than Data, is Finance (and sometimes Marketing, but usually to give numbers to Finance). Finance are unlikely to prioritise major resource spend on anything other than statutory requirements. This is why Finance will spend big on an ERP system but not on data - it's not the top priority.
Just because this order is in what I think is value order, doesn’t mean everyone should aim for the top. If you come from a Finance background or are that way inclined, 4 may be the kind of data work you get the most fulfilment from.
If you want to have the most impact in data possible, go work for a company who sell a data product in the division that makes it. This work will feel much more like engineering though - the exploratory investigative data work is usually not in 1 and instead is most likely to be in 2 and 4. I've had the privilege of speaking to data team leads, data product managers and founders doing 1 recently. I can't help but feel they are the ones living their best data lives and the ones focused on 4 are the embattled ones.
If you work in data and aren’t doing 1 to 4, and aren’t working in data platform… change what you’re doing.